

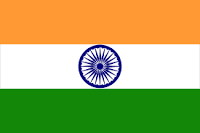

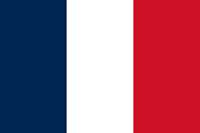




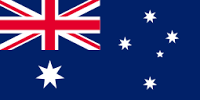


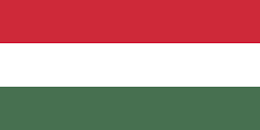


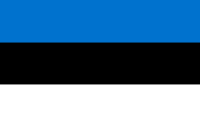



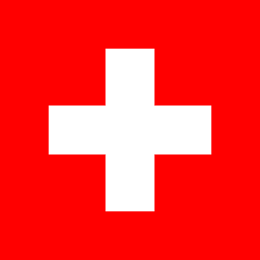




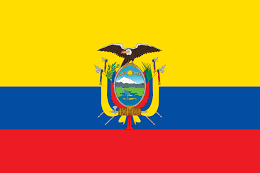

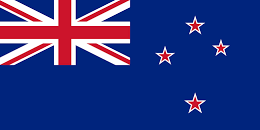

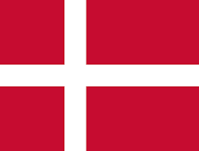

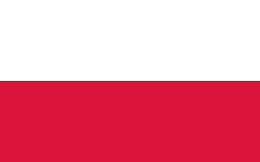

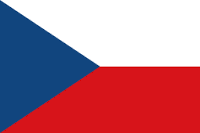

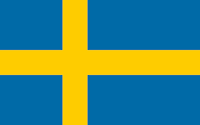


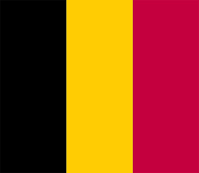

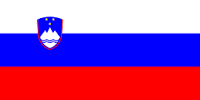


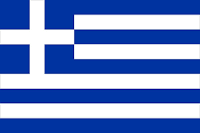
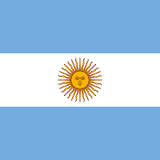
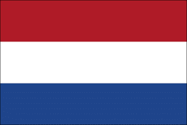




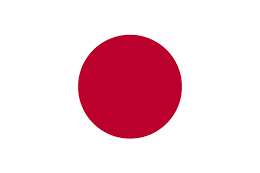

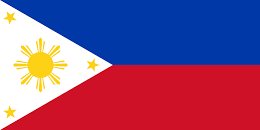


👋 Hello ,
🗞️Welcome to DataPro #123 – Your Weekly Data Science & ML Wizardry! 🌟
Keep up with the latest AI and ML insights, tools, and strategies to power up your projects. This week, we’ve curated the most exciting updates and resources to sharpen your skills and boost your results. Let’s jump in!
🧠 Algorithm Spotlight: Unlock the Tech Behind the Magic
◘ Veo and Imagen 3 on Vertex AI: Explore cutting-edge generative models.
◘ MarS Engine: Unified simulation for financial markets with generative AI.
◘ Run-Time Strategies for Next-Gen Models: A peek into advanced methods.
◘ MatterSimV1-1M & V1-5M: Microsoft’s latest open-source tools for AI research.
◘ Meet MegaParse: Open-source tool to prep documents for large language models.
◘ Promptwright by Stacklock: Create synthetic datasets with LLMs.
◘ Amazon Nova: High-performance foundation models for transformative AI.
🚀 Hot Trends: What’s Buzzing in AI & ML?
◘ Gemini for Restaurants: AI-driven operational insights for eateries.
◘ ML in Legacy Systems: Seamlessly integrate AI into your software.
◘ The Void IDE: Open-source AI for coding with precision.
◘ Top 10 Reinforcement Learning Repos: Master the art of RL.
◘ Python Tips: Tackle large datasets like a pro.
◘ Cross-Lingual Transfer: mBERT tricks for multilingual tasks.
◘ Amazon SageMaker Lakehouse: Simplify enterprise data management.
🛠️ Tools of the Trade: Pick the Best for Your Projects
◘ Fireworks.ai: Efficiency-first generative AI engine.
◘ Amazon Q Developer: Modernize mainframes with generative agents.
◘ Matrix Transformations Explained: A guide to interpreting matrix math.
◘ Univariate Exemplar Recommenders: Customer profiling, simplified.
◘ SQL vs. Calculators: DIY champion/challenger tests.
◘ Google Colab Tips: Train language models with ease.
◘ PostgreSQL Optimization: Smarter queries for everyday use.
📊 Real Wins: Learning from Case Studies
◘ Data Science Journeys: Lessons from experienced practitioners.
◘ RAG Systems: Exploring Retrieval-Augmented Generation.
◘ Prompt Engineering Expertise: Build skills that matter.
◘ ML Experiments Done Right: Best practices for experimentation.
◘ Model Validation: Techniques for robust evaluations.
◘ Explainable Recommendations: Making AI in news more transparent.
◘ Enterprise AI Chatbots: Why they fail and how to fix them.
Enjoy exploring, learning, and building this week!
Stay tuned and stay inspired – there’s always something new to discover in the ever-evolving world of Data Science and Machine Learning!
Take our weekly survey and get a free PDF copy of our best-selling book,"Interactive Data Visualization with Python - Second Edition."We appreciate your input and hope you enjoy the book!
Share Your Insights and Shine! 🌟💬
Cheers,
Merlyn Shelley,
Editor-in-Chief, Packt.
This 3 hour power packed workshop that will teach you 30+ AI Tools, make you a master of prompting & talk about hacks, strategies & secrets that only the top 1% know of.
By the way, here’s sneak peek into what’s inside the training:
- Making money using AI 💰
- The latest AI developments, like GPT o1 🤖
- Creating an AI clone of yourself, that functions exactly like YOU 🫵
- 10 BRAND new AI tools to automate your work & cut work time by 50% ⏱️
1.5 Million people are already RAVING about this hands-on Training on AI Tools. Don’t take our word for it? Attend for yourself and see.
Sponsored
➽ RAG-Driven Generative AI: This new title, RAG-Driven Generative AI, is perfect for engineers and database developers looking to build AI systems that give accurate, reliable answers by connecting responses to their source documents. It helps you reduce hallucinations, balance cost and performance, and improve accuracy using real-time feedback and tools like Pinecone and Deep Lake. By the end, you’ll know how to design AI that makes smart decisions based on real-world data—perfect for scaling projects and staying competitive! Start your free trial for access, renewing at $19.99/month.
➽ Building Production-Grade Web Applications with Supabase: This new book is all about helping you master Supabase and Next.js to build scalable, secure web apps. It’s perfect for solving tech challenges like real-time data handling, file storage, and enhancing app security. You'll even learn how to automate tasks and work with multi-tenant systems, making your projects more efficient. By the end, you'll be a Supabase pro! Start your free trial for access, renewing at $19.99/month.
➽ Python Data Cleaning and Preparation Best Practices: This new book is a great guide for improving data quality and handling. It helps solve common tech issues like messy, incomplete data and missing out on insights from unstructured data. You’ll learn how to clean, validate, and transform both structured and unstructured data—think text, images, and audio—making your data pipelines reliable and your results more meaningful. Perfect for sharpening your data skills! Start your free trial for access, renewing at $19.99/month.
⫸ Introducing Veo and Imagen 3 on Vertex A: This blog highlights Google Cloud's transformative generative AI tools, Veo and Imagen 3, on Vertex AI, enabling businesses to create high-quality videos and images effortlessly, reduce production costs, and unlock creative potential while ensuring safety and responsibility.
⫸ MarS: A unified financial market simulation engine in the era of generative foundation models: Microsoft Research is advancing financial market analysis with MarS, a simulation engine powered by generative foundation models. By leveraging domain-specific financial data, MarS enables enhanced efficiency, insights, and adaptability for tasks like market prediction, risk assessment, and trading strategies.
⫸ Advances in run-time strategies for next-generation foundation models: This blog explores advancements in frontier language models, highlighting OpenAI’s o1-preview achieving 96% accuracy on MedQA, outperforming GPT-4 with Medprompt. It examines run-time strategies, cost-efficiency, and prompting techniques for improving performance in medical challenge benchmarks.
⫸ Microsoft Released MatterSimV1-1M and MatterSimV1-5M on GitHub: Microsoft's MatterSimV1-1M and MatterSimV1-5M, now on GitHub, revolutionize materials science with deep-learning models for precise, rapid simulations across diverse conditions. These tools predict properties like phase stability and Gibbs free energy, accelerating material discovery and engineering.
⫸ Meet MegaParse: An Open-Source AI Tool for Parsing Various Types of Documents for LLM Ingestion. MegaParse is an open-source tool streamlining document preparation for large language models (LLMs). It supports diverse formats like PDFs, Word, and Excel, retaining data integrity while automating conversion into LLM-ready formats for efficient and accurate AI-driven workflows.
⫸ Stacklock Releases Promptwright: A Python Library for Synthetic Dataset Generation Using an LLM (Local or Hosted). Promptwright, Stacklock's new Python library, simplifies synthetic dataset generation using local or hosted LLMs like OpenAI, Anthropic, and Gemini. It empowers developers with customizable prompts, multi-provider support, and seamless Hugging Face integration, bridging data gaps efficiently for AI projects.
⫸ Amazon Introduces Amazon Nova: A New Generation of SOTA Foundation Models that Deliver Frontier Intelligence and Industry Leading Price-Performance. Amazon Nova redefines foundation models with versatile, cost-effective AI solutions via Amazon Bedrock. From text-only Micro to multimodal Pro, it balances scalability, affordability, and performance, offering extended context handling, fine-tuning, and robust global accessibility for diverse business needs.
⫸ Use Gemini to optimize restaurant operations through AI visual analysis: Gemini 1.5 Pro revolutionizes business operations with multimodal AI and long-context window capabilities. From inventory management to safety assessments, it enables efficient AI-powered insights such as real-time kitchen analysis for restaurants, boosting productivity, training, and workplace safety.
⫸ Integrating Machine Learning into Existing Software Systems: This blog explores key concepts, tools, and strategies for integrating machine learning models into existing software systems, addressing challenges like scalability, compatibility, and cost, while highlighting frameworks, containerization tools, MLOps platforms, and cloud solutions for seamless implementation.
⫸ Enter The Void: An Open Source AI Coding IDE. This blog introduces Void, an open-source AI-powered code editor positioned as a community-driven alternative to Cursor. It highlights Void's features, customization capabilities, and steps for building the IDE locally, empowering developers to create and innovate independently.
⫸ 10 GitHub Repositories to Master Reinforcement Learning: This blog highlights 10 GitHub repositories to master reinforcement learning, offering free resources, including tutorials, projects, and algorithms. It’s a practical guide for learners to explore RL concepts, apply them through projects, and stay updated on the latest trends.
⫸ Tips for Handling Large Datasets in Python: This blog provides practical tips and tools for handling large datasets in Python, including memory-efficient techniques, parallel and distributed computing with Dask and PySpark, and chunked processing with Pandas to streamline big data workflows.
⫸ How to Implement Cross-Lingual Transfer Learning with mBERT in Hugging Face Transformers? This article explains how to fine-tune the multilingual BERT (mBERT) model from Hugging Face for cross-lingual transfer learning, showcasing its ability to generalize across languages by training on English data and evaluating on French datasets.
⫸ Simplify data access for your enterprise using Amazon SageMaker Lakehouse: This article explains how to use Amazon SageMaker Lakehouse to unify data from warehouses and lakes, enabling secure, scalable analytics and machine learning for businesses. It showcases a case study on customer churn prediction and provides a step-by-step implementation guide.
⫸ Fireworks.ai: Lighting up gen AI through a more efficient inference engine: This blog introduces Fireworks AI, an advanced gen AI inference engine designed to help enterprises scale, optimize costs, and deploy AI models efficiently. It highlights Fireworks’ collaboration with Google Cloud and NVIDIA to deliver cutting-edge, scalable, and secure AI solutions.
⫸ Simplify Mainframe Modernization using Amazon Q Developer generative AI Agents: This blog introduces Amazon Q Developer, a generative AI-powered solution for mainframe modernization. It automates code analysis, planning, and refactoring, enabling faster, cost-effective transitions to cloud-native architectures while preserving critical application logic and improving agility, security, and scalability.
⫸ How to Interpret Matrix Expressions—Transformations? This article is the first in a series designed to simplify matrix algebra for data scientists. It focuses on interpreting complex matrix expressions, providing intuitive, practical explanations of key concepts like transformations, transposition, and inverses, with a focus on machine learning applications.
⫸ Introducing Univariate Exemplar Recommenders: how to profile Customer Behavior in a single vector: This blog explores exemplar recommenders, a vector-based architecture for recommendation systems that enhances scalability and accuracy. It introduces multivariate and univariate approaches, highlights clustering methods, and focuses on improving recommendation variance while addressing computational challenges in user preference profiling.
⫸ SQL vs. Calculators: Building Champion/Challenger Tests from Scratch. This blog explores the transformative power of champion-challenger testing (A/B testing) in business decision-making, using SQL for implementation. It discusses the $300 million button case, test setup, key metrics, and sample size calculations to optimize strategies and drive measurable results.
⫸ Training Language Models on Google Colab: This blog provides a guide to fine-tuning large language models on Google Colab efficiently. It addresses Colab's limitations by utilizing Google Drive for saving checkpoints, enabling resumption of interrupted training, and offers reusable code for persistent experimentation across sessions.
⫸ PostgreSQL: Query Optimization for Mere Humans. This blog explores how to optimize SQL queries by leveraging PostgreSQL's EXPLAIN and EXPLAIN ANALYZE clauses. It demystifies execution plans, identifying bottlenecks, and improving database performance with practical tips and a deep dive into execution plan anatomy.
⫸ Becoming a Data Scientist: What I Wish I Knew Before Starting. This blog outlines a practical roadmap for aspiring data scientists, emphasizing foundational skills in mathematics, programming, SQL, and machine learning. It stresses business impact, focusing on the Pareto Principle, and encourages hands-on experience to transition effectively into the data science field.
⫸ From Retrieval to Intelligence: Exploring RAG, Agent+RAG, and Evaluation with TruLens. This blog explores enhancing Large Language Models using Retrieval Augmented Generation (RAG) with LlamaIndex, addressing limitations in detail specificity and outdated knowledge, while integrating TruLens for performance metrics and emphasizing efficient, expert-like responses over extensive web searches.
⫸ How to Build Prompt Engineering Expertise at Your Company? This post explores whether companies should hire dedicated prompt engineers or grow this expertise internally, highlighting the role’s evolving nature, necessary skills like creativity and curiosity, and strategies for nurturing prompt engineering talent to leverage generative AI effectively.
⫸ Machine Learning Experiments Done Right: This post outlines a detailed checklist for conducting rigorous, reproducible machine learning experiments, addressing design, data selection, systematic testing, and cross-validation to ensure valid and reliable results, while avoiding common pitfalls like data contamination and misreporting.
⫸ Model Validation Techniques: This post explains 12 model validation techniques for testing machine learning model reliability, showcasing their evolution and distinctions through a consistent dataset example, focusing on practical applications and why choosing the right method matters.
⫸ Making News Recommendations Explainable with Large Language Models: This post explores the use of Large Language Models (LLMs) for news article recommendation at DER SPIEGEL, highlighting their predictive accuracy, explainability, and potential to enhance user engagement. Challenges include high costs, slow processing, and optimization opportunities for improved scalability.
⫸ Why Internal Company Chatbots Fail and How to Use Generative AI in Enterprise with Impact? This article highlights a process-driven approach to generative AI in enterprises, emphasizing AI process orchestration over chatbots. It discusses designing structured workflows with reusable templates to improve reproducibility, efficiency, and quality, avoiding over-reliance on inconsistent chatbot interactions.